
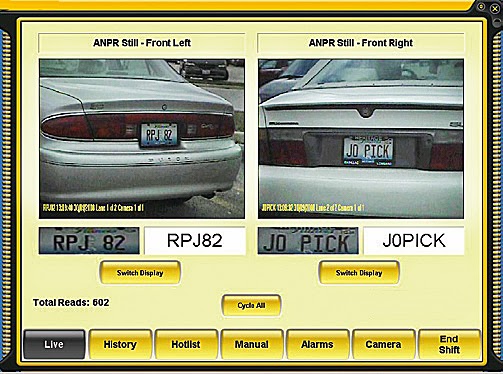
Each layer has been related with interconnection weights.

In this method, there are 3 layers namely input, hidden, and output layers has been utilized. The number plate is detected using a multi layered feed forward back propagation neural network (MLFFBPNN). Deep learning-based license plate identification using neural networks is proposed in this article. The use of machine learning and deep learning algorithms, which display improved classification accuracy and resilience, has been a significant recent breakthrough. Vehicle license plate identification is still a challenge with current approaches, particularly in more complicated settings. Real-world traffic situations, including smart traffic monitoring, automated parking systems, and car services are increasingly using vehicle license detection systems (VLDS). The research results show that the network has accurate license plate recognition and strong robustness in complex scenes. The experimental results show that the network has an average recognition rate of 99.3% on the CCPD public license plate dataset and up to 99.2% on the self-built XAUAT-Parking dataset. The proposed network performs license plate recognition accuracy experiments on both the self-built XAUAT-Parking dataset and the publicly available CCPD dataset. Finally, a multi-scale semantic license plate character extraction module is proposed to decode and recognize the character features in the reconstructed license plate image. Second, in the absence of license plate character location label information, the license plate characters and background in the global feature map are decoupled using a multi-scale region attention mechanism to progressively weight different regions of theįeature map at different scales to improve the network's ability to focus on the saliency of character regions and the anti-interference ability of background noise regions. Key character features in distorted and blurred low-resolution license plate images are reconstructed. By introducing mutual information constraints on color distribution and character structure features, the discriminative ability of the network on different feature dimensions is improved. First, a multi-scale regional attention license plate super-resolution module is proposed based on the InfoGAN framework.
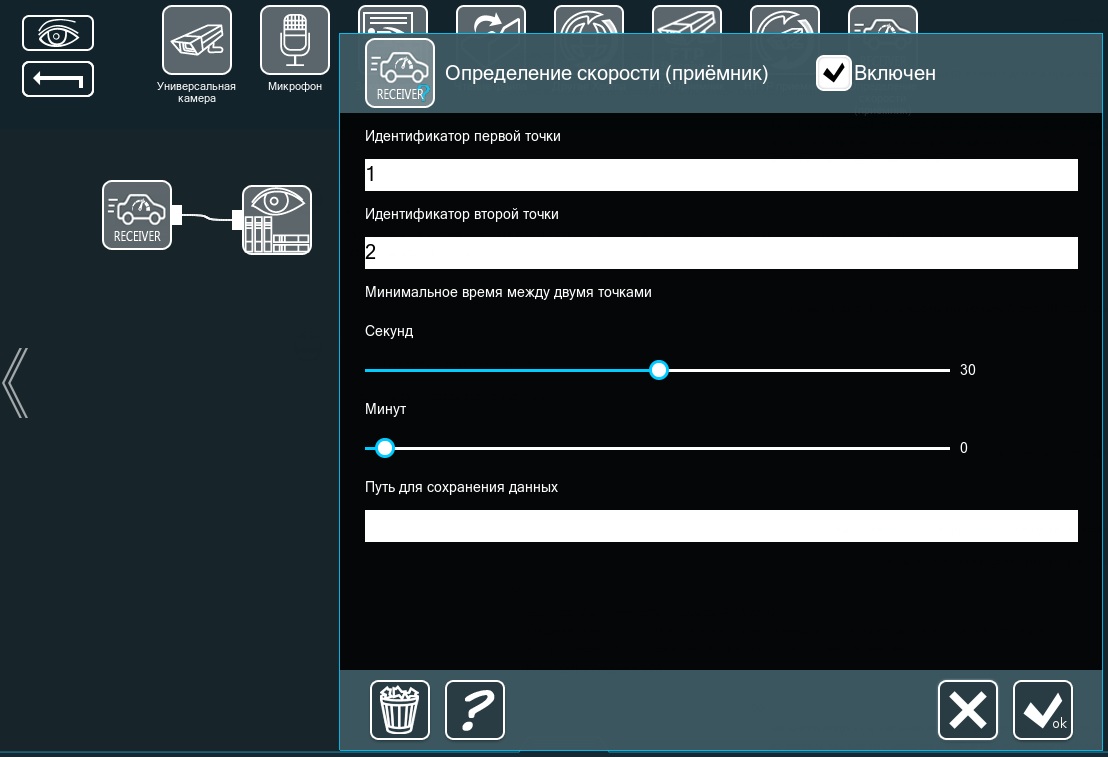
In CCPD-Base, CCPD-DB, CCPD-FN, CCPD-Tilt, CCPD-Weather, CCPD-Challenge and other sub-datasets, the recognition rates reach 99.3%, 98.5%, 98.6%, 96.4%, 99.3% and 86.6% respectively.Ībstract: A multi-scale regional attention InfoGAN license plate recognition network is proposed for the problem of difficult recognition of license plate images caused by skewing, obscuring, distortion and blurring of license plate images. A large number of experimental results demonstrate that the proposed algorithm has good performance under unrestricted conditions, which proves the effectiveness and robustness of the model. Finally, 1D-Attention is adopted to enhance useful character features after Bi-LSTM positioning, and reduce useless character features to realize effective acquisition of character features of license plates. Then locate each license plate character through Bi-LSTM combined with the context location information of license plates. First of all, the model can activate the regional features of characters and fully extract the character features of license plates. In order to improve the accuracy of license plate recognition under unrestricted conditions, a robust license plate recognition model is proposed in this paper, which mainly includes license plate feature extraction, license plate character localization, and feature extraction of characters. from the Chinese City Parking Dataset (CCPD), the performance of some methods of license plate recognition will be significantly reduced. However, for license plates under non-restrictive conditions, such as dark, bright, rotated conditions etc. At present, most methods have favorable effect on license plate recognition under restrictive conditions, and most of such license plates are shot under good angle and light conditions. License plate detection and recognition are still important and challenging tasks in natural scenes.
